Report: Put small cells “where there is high traffic demand but low signal quality”
Small cell deployments have followed a few broad trends in the past few years. The first wave serve to fill in holes in network coverage; the next wave was used to augment capacity in geographic bottlenecks. The third, and ongoing wave, is partly a continuation of the second wave but bolstered by the sheer density requirements of 5G, particularly 5G that uses millimeter wave frequencies.
Given this paradigm, coupled with the typical site acquisition, backhaul, power, rent issues associated with small cells, operators need to locate small cells in a manner that maximizes return on investment. As highlighted in a recent white paper from 5G Americas and the Small Cell Forum titled “Precision planning for 5G era networks with small cells,” maximum ROI comes when small cells are “placed as close as possible to demand peaks; best practice is within 20-40” meters.
Download the full white paper here.
So, if best practice is place a small cell 120-feet at most from where demand peaks but isn’t adequately served by the existing network, how are operators supposed to figure that out? According to the report authors, machine learning is key.
In detailing other best practices in optimizing small cell placement, the white paper authors specify:
- “MNOs would like equipment that estimates location of usage and quality reports to adopt smarter algorithms such as the machine learning approach demonstrated. Median locate errors less than 20 [meters]are expected for small cell planning purposes.
- “Machine learning models should be part of any small cell design effort. Different inputs and assumptions will be factors in the resulting models that are generated.”
In order to deliver capacity where it’s needed, operators need a good baseline data set of where user equipment is. The report authors suggest two-dimensional mapping is no longer adequate as it doesn’t take into account where network load in a multi-story building is originating; hence, 3-D mapping is an underlying planning requirement.
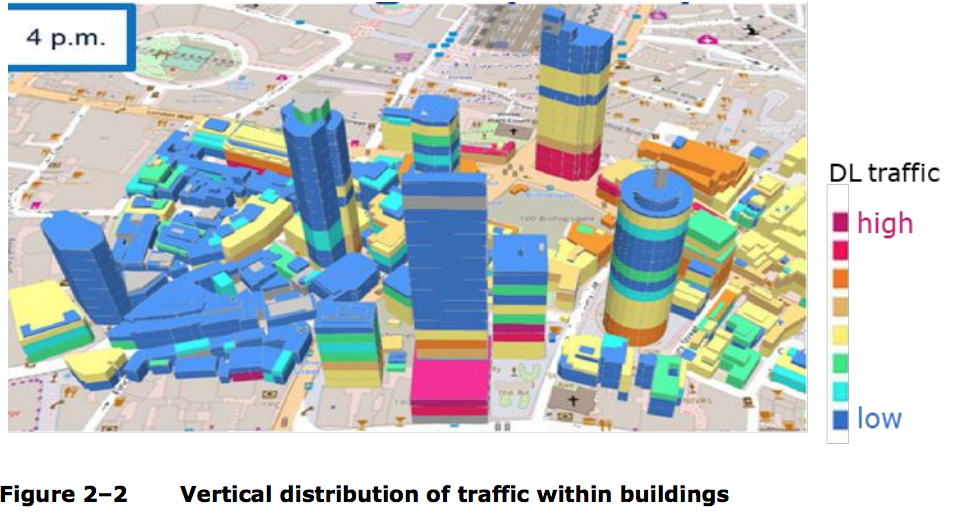
Image courtesy of Small Cell Forum/5G Americas.
That data is feed in a software model that also includes things like sector count, azimuth, fiber availability, street furniture, fronthaul and backhaul and considerations around the frequency or frequencies the operator plans to use in a specific location, according to the report.
That’s where machine learning comes into play. The authors recommend, “Data is added to the model, including terrain and structures in the area, and the associated clutter classes and heights. Assumptions are made regarding the antennas (omni-directional, brand, etc.) Candidates are generated and classified and factored in the following priority order: 1. Existing LTE assets 2. Locations where we have agreement with site owners 3. Intersections identified by open street maps 4. Uniform hexagonal grid.”
When an ML approach is compared to a more manual network design process, the report finds the former resulted in 111 sites over an area the size of Manhattan compared to 185 sites coming out of the latter process. That has a direct line to both capex and opex.
According to Nokia’s 5G Principal Architect Peter Love, 5G will force operators to become “digital service providers. By using big data analytics, including machine learning, to digitally model specific use cases, will deliver better returns on investment for network evolution plans and hence a better business outcome.”
The post How machine learning can improve siting for small cells appeared first on RCR Wireless News.